4 minute read
Machine learning: Seeing behind the curtain
Artificial intelligence. It’s an idea that excites and inspires many, as much as it concerns others. But it’s already here, making a difference. In the age of Industry 4.0, it gives us the opportunity to do things better, and smarter – we just need to see the potential behind the curtain. Richard Chamberlain from Bosch Rexroth explains that up to now, we’ve been asking the wrong question: will machines become more intelligent than us? We should be asking: how will intelligent machines help us become more intelligent? The key lies in transparency of data – fundamental to the concept of machine learning.
With transparency in mind, let’s go right back to the start. What exactly is machine learning? Very simply, it’s a field of computer science in which software is given the ability to solve complex problems, undertake increasingly complex processes and tasks, and crucially, learn.
Another question would be, where does machine learning fit into the context of artificial intelligence? The simple answer here: it is AI. Machine learning software starts – essentially – as an infant; exploring its systems and surroundings, learning pathways and protocols, before learning ever-more efficient ways to achieve tasks and solve complex problems. The machine never stops learning. A learning machine is AI, created within set parameters for a purpose that benefits us – whether it’s an e-commerce platform that learns what a customer likes (“because you liked X, we thought you might like Y”), or an industrial maintenance routine keeping machines moving. So, again in the spirit of transparency, now we know what it is, how would we go about making it happen?
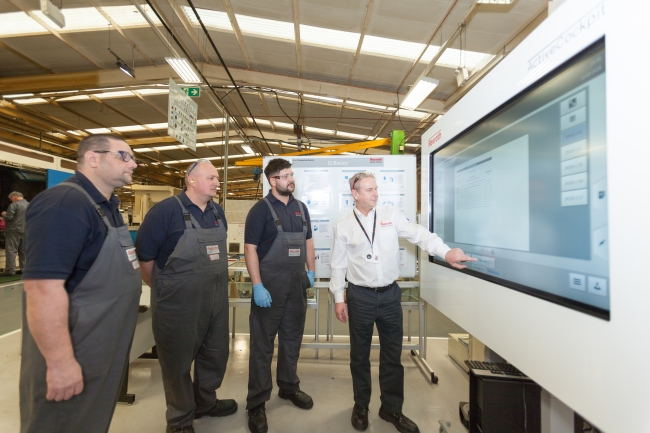
Manufacturers should be asking: how will intelligent machines help us become more intelligent? / Picture: Bosch Rexroth
Built on data
Our world is being shifted by big data. It’s core to the connectivity that links us – and to machine learning. The sensors at the core of Industry 4.0 collect huge, comprehensive data sets that make it all possible – but data without intelligence is not enough. It’s what you do with it that counts. There’s a cyclical, three-step process by which data is rendered useful: visualise, evaluate, act. Firstly, we visualise the data, and evaluate its patterns and purpose. Then, and most importantly, we act on the data, to change systems in line with findings – then repeat. It’s an iterative process like many fields outside computer science, but it’s critical to giving big data a real purpose.
This is made possible by the other main ingredients of machine learning: algorithms and mathematical models. These are written to make best use of the data; even down to each sensor having an algorithm and model with set parameters. From these bases, combining huge computing power with precision training, software learns to use this data for itself, to see and connect patterns, pathways and predictions; to visualise, evaluate, act, improve and learn.
On a side note, it’s worth remembering the valid concerns people have about data collection, storage and usage – especially given stories dominating the news right now. A key point here – again – is transparency. Giving customers confidence in how their data will be collected, stored and used is paramount. Bosch Rexroth, for example, offers data storage offsite, on a secure server in Europe. Customers have full and free visibility and control over their data.
Theory, practically applied
We’ve seen what’s behind machine learning – but where would you find it in the real world? As previously stated, it’s at work on social media and e-commerce, as software learns who you are, who you know – and might know – and what kind of products you may like. But what about within industry? How are organisations applying machine learning, and why?
Once again, it’s all about transparency, giving customers visibility and control over their data and, in turn, delivering three benefits: reducing costs, increasing quality, and increasing output. To achieve these, machine learning can take on three forms and functions: condition monitoring, predictive maintenance and quality management.
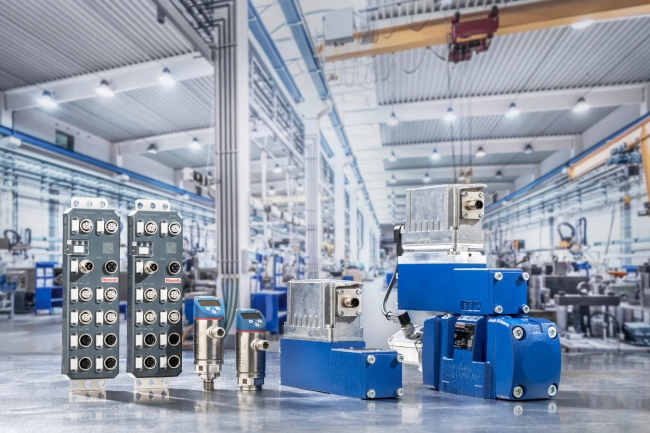
Software learns to use this data for itself, to see and connect patterns, pathways and predictions; to visualise, evaluate, act, improve and learn / Picture: Bosch Rexroth
Let’s look at an example: a ‘health index’ program for predictive maintenance. This combines data, algorithms and models into software that can proactively monitor and predict asset condition. Factoring in multiple variables, observing usage patterns and making relevant connections, it can alert the customer when asset health will degrade past a threshold. One key advantage here is in the accuracy of fault-finding and prediction. In a given case, statistically speaking, detecting a fault by chance only carries a probability of 13%. Expert human monitoring still only achieves a success rate of around 43%. But machine learning increases fault detection to over 95% – granting greater transparency over the long-term health of equipment, and reducing costs while improving quality and output.
Machine intelligence starts with human intelligence
The possibilities for machine learning in industry are limitless – so, how can you get started? The most important factor is finding the right partner to work with. Data scientists who gather, visualise, evaluate and act on data are essential, but there’s a wider picture too. It’s not just software; hardware, sensors and technology need to be connected in an end-to-end system – so a single-source, turnkey solution is ideal. Similarly, flexibility of service to build systems for specific needs is vital, just as is the experience and expertise to solve problems and pain points, and apply relevant use cases to deliver the right solution.
Just as machine learning is built on data, artificial intelligence starts with human intelligence. That’s the truth behind the curtain – the real transparency. But the value of machine learning cannot be overstated in our connected world. The smarter our software gets, the smarter we can work – and the smarter we can work, the greater the benefits for our industries, our lives and our world.