4 minute read
Making data; where next for performance management in manufacturing?
Manufacturing businesses are all about three things; low overheads and raw material costs, continuously repeatable process, and consistently high-quality products; get these right and the rest will follow. Dan Burrows from Waterstons looks at how effective management of this kind of business relies on timely, accurate information to drive decision making and identify opportunities for efficiency-improvement; information which supports the continuous improvement agenda of modern manufacturing.
Buzzword-bingo in the manufacturing boardroom is enlivened by the addition of ‘Lean’, ‘Kaizen’, ‘Six-Sigma’, ‘Deming’, ‘Thrift’ and all of the other terms and words we use to describe the fundamentals of what may sound like common sense; doing more with less. Mass manufacturing has been improving efficiency through the reduction of waste for as long as it has existed; whether saving wasted time through automation of the weavers’ loom or more recently reducing stockholding via more tightly controlled supply chains, there’s nothing new under the sun.
What we do have is the proven effectiveness of some of the collected wisdom of the ‘improvement pioneers’, as demonstrated by the methodologies adopted by some of the world’s largest manufacturers, from Toyota to Toshiba and Fujitsu to Ford. Each with their own way of implementing continuous improvements, the principles remain similar and supporting those principles relies on knowledge and understanding. One cannot make something better unless one understands where and why it is not at its best; if knowledge is power, information is its battery.
Over recent years, business intelligence, analytics and performance management have been at the forefront of the information agenda; with the advent of integrated systems within businesses there has been presented the opportunity to consolidate sources of data, reduce manual manipulation and intervention in reporting, review and refine the measures used to track performance, efficiency and capacity and create personalised views of the information world. Business leaders and managers have greater quantities and higher quality of data than ever before, and where they are able to exploit it to its greatest potential the rewards can be significant.
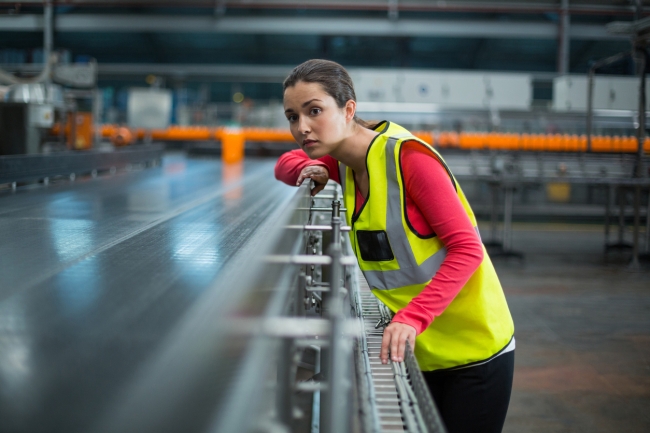
Manufacturers should embrace the opportunity to anticipate and predict inefficiency in their factories / Picture: Getty/iStock
Oft-untapped, however, is a source of information which can fill in another piece of the jigsaw. Improving the way one does things now is all very well, but what of the opportunity to anticipate and predict inefficiency? What if one could anticipate production problems, equipment failures and maintenance requirements?
Modern processing and manufacturing equipment, from the simplest conveyor-belt to the most complex multi-stage robotic assembly line, relies heavily on computerised control. PLC (programmable logic controller) systems can manage and maintain every element of the modern factory floor, monitoring everything from cooking temperatures to bolt torque and vibration to alignment; there is virtually nothing a computer can’t do faster and more efficiently than a human when it comes to looking after a machine.
Data volumes generated by sensors and control systems can be prodigious; as an example, information from CERN tells us that the Large Hadron Collider’s many sensors and control systems generate over a petabyte (one million gigabytes, or 62,500 iPhones’-worth) of data every second. Luckily for CERN, they can filter that down to a more manageable one gigabyte per second, but that’s still… well, quite a lot.
Obviously, a conveyor belt doesn’t hold the keys to the secrets of sub-atomic physics, so the challenge you face won’t be quite on the same scale; but it’s still generating a lot of information and you will still need to pick and choose what you want to store. That may mean sampling its speed every hour instead of every minute, or maintaining only a time-limited subset of the data.
Exploiting this information needn’t be complicated; monitoring a machine’s efficiency with the goal of predicting maintenance requirements or anticipating failures can often be as simple as noticing early enough that a key parameter of its operation has drifted away from a nominal value.
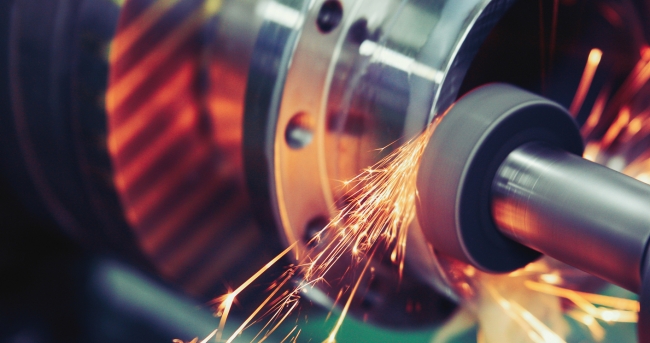
Performance management of assets in a factory needn’t be complicated / Picture: Getty/iStock
A bearing running hotter than normal, increased vibration in a shaft assembly, a decrease in hydraulic pressure; all indicators that something’s not quite as it should be. Small things can have large consequences and it’s better to stop the line for an hour to investigate and fix a small problem than to lose a day’s production due to catastrophic failure: For want of a nail the shoe was lost…
Solutions to monitor performance can be complicated by the requirement to select a baseline value for comparison; for example, making the assumption that a bearing runs at 75°c and monitoring its temperature against that target. However, environmental conditions, differences in installation and construction, and other peculiarities particular to an individual machine mean that it can be difficult to establish accurately the target values.
A far more simplistic approach is to baseline performance against a continuously calculated rolling average; the monitor takes periodic readings and the average value in a defined period is calculated. A threshold is set and the monitor immediately flags any situation in which readings over a period are greater or less than the nominal average calculated.
Manufacturers must recognise and exploit the value of this type of information; as in any business, data is an important asset and the way in which it is used can mean the difference between success and failure. Whatever the output of the manufacturing process, one of the by-products is the information required to create it.
The same principles that are applied to reducing wasted time and materials can equally be used to reduce waste of useful data, improving decision making still further and assisting with addressing the three goals with which I opened; optimising maintenance and repair spend, improving process reliability and speed, and ultimately supporting the delivery of high-quality, low-cost-to-make products.