3 minute read • published in partnership with Cimlogic
Insight: How can machine learning solve your quality challenges?
The cost of quality issues in batch processes can be significant. Especially if they result in the loss of an entire batch, which could have been hours or even days in the making. Often batch processes involve multiple moving parts: quantities, temperatures, pressure, agitation, time and operator interaction. Steve Wilkinson, chief technical officer at Cimlogic shares batch process improvements discovered during a recent client project.
The cost of quality issues in batch processes can be significant. Especially if they result in the loss of an entire batch, which could have been hours or even days in the making. Often batch processes involve multiple moving parts: quantities, temperatures, pressure, agitation, time and operator interaction.
While most manufacturers understand how each one of these can adversely affect the batch on its own, it becomes much harder to understand the likely impact on quality when each of them is changing at the same time.
It’s not unheard of to experience a batch where all individual parameters were within tolerance – but still, the final outcome was not as expected. Was it down to each variable being near tolerance at the same time? A slight difference in timing of steps? It can be hard to understand from trends alone.
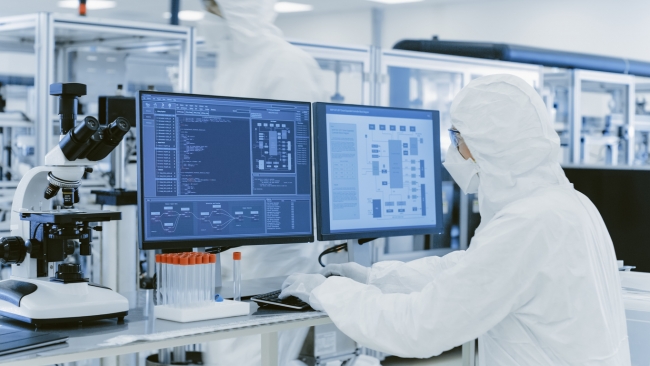
Machine learning techniques help identify process deviations to help aid root cause analysis and help solve quality challenges / Picture: Getty/iStock
Using machine learning, Cimlogic is able to build models that can understand these complex relationships, learn from the outcome of historical batches, and what combination of factors result in good or bad quality.
In a recent study of a client’s data, Cimlogic were able to build a model that could identify a number of key events that were critical to the quality of the batch:
1 – Missed or poorly timed step execution
By looking historically at several good batches, Cimlogic were able to train a machine learning model that could spot differences in the active batch that suggested it was going off-course. In this case, the model could detect the impact of an important step that could be missed by the operator. The steps in question were performed manually and without automation or instrumentation, meaning there was no obvious way in the current SCADA to detect if the step had been performed or not.
Using Cimlogic’s machine learning model, we were able to identify (through the behaviour of the batch process variables), and measure, the likelihood that a step has been missed. As time progresses through the batch, the model becomes more ‘certain’ that the step is missed – and after a determined threshold can alert the operator. This allows them to perform the step soon enough that the batch can be saved – removing those mistakes that are expensive in both lost time, materials and opportunity.
2 – Batch Success Prediction
Cimlogic created an analytical model that could detect the specific characteristics of a batch, to indicate that the batch was nearing successful completion. Due to the nature of this particular batch process, the duration of the batch was hard to predict and dependent on many variables.
By understanding the properties of a batch and its outcome historically, the model was able to provide an early indication that that batch would be successful. This alert allowed the downstream processes to begin preparation of their time-sensitive steps. The result? Reduced waste accrual through preparing the downstream process too early or too late. Too early and it must be repeated, too late and the batch must be scrapped. For Cimlogic’s client – this was a significant saving.
What’s the value of predicting?
Early indication of batch outcome can be extremely valuable – perhaps you have a downstream process that must wait for the validation of some lab results. The same process can be applied to give a ‘positive pre-release’ of batches – releasing them on a defined confidence level, allowing production to continue while lab results are validated.
Machine learning techniques such as the examples above help identify process deviations to help aid root cause analysis. By predicting if a batch is likely to fail and allowing operators and managers to take corrective action, wasted product and time can be avoided – ensuring product quality is maintained and waste is reduced.