4 minute read • published in partnership with Cimlogic
Insight: Machine learning for performance management
“Manufacturing: it’s so predictable!” It’s probably not a phrase we use that often when faced with unplanned events such as breakdowns or quality issues. It’s easy to feel like Manufacturing is highly unpredictable. Steve Wilkinson, chief technical officer at Cimlogic shares his view on how manufacturers can embrace machine learning to support improved performance and productivity.
When we step out of those day-to-day issues we know, of course, that predictability and consistency are exactly what we are trying to achieve. So why, after all that hard work designing a repeatable process for manufacturing and trying to eliminate the root cause of downtime and quality issues do we still feel like manufacturing is hard to predict? Well, as we strive for shorter cycle time, higher production volumes and lower costs, we challenge the steady-state that we have been trying to create. Throw the human element into that mix as well and we have a recipe for randomness.
As humans, we can make sense of the variables that might affect a process. An experienced operator can look at a production plan or a process trend and make a judgement call about the problems they are likely to experience that day. Like whether they are likely to finish on time, or be asked to do overtime. However, again as humans, we can only process a handful of variables at any one time and we base our predictions on our past experiences. As none of us can work 24/7 the predictions of one person will likely be different to that of another.
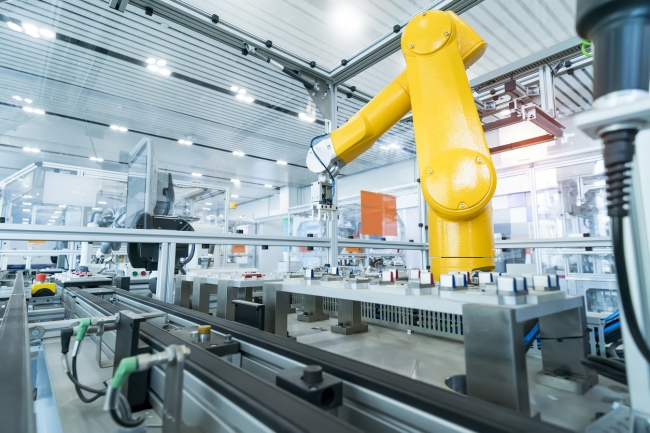
By embracing machine learning manufacturers can improve performance and productivity at factory sites / Picture: Getty/iStock
When you consider other factors such as people, differing operating procedures, machine health, raw material variability, storage and movement conditions, and environmental changes such as weather, the number of variables grows and human-predictability begins to drop off. This is where the reliance on gut decisions begins to increase. Gut decisions are those where we cannot easily explain the rationale. Gut decisions are still based on experience and in fact, may be the result of combining a lot of inputs and experiences subconsciously and creating a best guess. They are not the same as a lucky guess. Therefore, you will likely find in a really experienced operator, that these gut decisions are actually pretty good. Unfortunately, the experienced workers are becoming scarce and the ones we do have are far too useful to be staring at trends all day!
Now, what if we could capture that same experience 24 hours a day 7 days a week, and do it for multiple variables, at faster speeds and for longer periods of time? What if we could do it without forgetting, and without bias or preconception?
In steps machine learning
Machine learning (ML), a subset of Artificial intelligence (AI), does exactly that. It is not scary or some kind of magic. In fact, (without wanting to undermine the incredible amount of academic research in the field) it’s just some very clever maths. ML has been around as a concept since the 1950s and some of the underpinning maths hundreds of years before that. What has happened recently is that ML has become more accessible, both in terms of the compute required to ‘train’ it, and in the abstraction of that clever maths. And finally, AI and ML are making it into the manufacturing space.
ML in manufacturing does nothing that our experienced worker from before could not do, but as machine learning is performed by a machine (the clue is in the name), it can do it faster and more reliably – in a way that would not be feasible to scale with people-power alone.
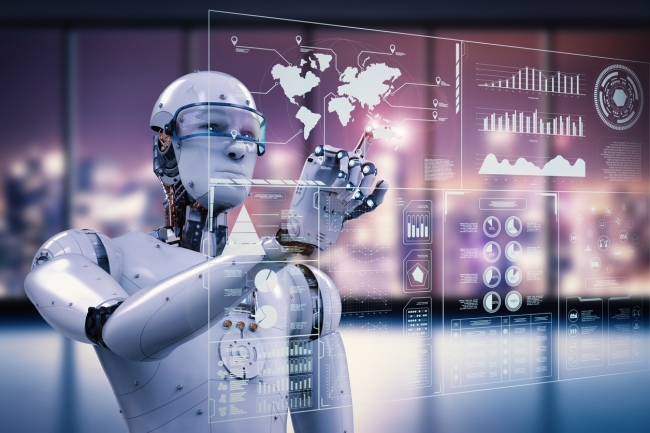
By understanding how you ‘normally’ run a plant, machine learning can spot changes before they become operational issues / Picture: Getty/iStock
So how does that help us in our strive for productivity?
Well, ML can:
• Support the decisions being made when the human experience is not on hand. By learning statistically from historical data, ML models can predict an outcome or variable that is dependent on the historical data it has consumed.
• Monitor your processes and spot anomalies which could be early indicators of impending issues. It can predict how likely you are to achieve the plan today, or tell you what common issues you should expect on the upcoming batch and help find their root causes.
• Predict more accurately your runtimes or your changeover times – allowing you to plan more accurately and in turn reduce unexpected costs such as labour or energy costs incurred from running an extra shift.
• By understanding how you ‘normally’ run a plant, it can spot missed steps or operator errors and enable action before it becomes an expensive operational issue.
It’s fair to say there are endless opportunities for machine learning in performance management, and I’m looking forward to sharing some of the improvements we are discovering in this space in future.