4 minute read • published in partnership with Cimlogic
Insight: Machine learning – the real business benefits across the manufacturing supply chain
When making the case for machine learning manufacturers need to identify the real business benefits across the supply chain. Steve Wilkinson, chief technical officer at Cimlogic shares some performance improvements discovered during recent client Machine Learning projects.
Utilising machine learning to predict maintenance events resulting from faulty equipment for improved batch quality
Left undetected, a failure in sensing equipment can result in significant quality issues – potentially reworking or even worse, scrapping batches that have been days in the making. As OT technologies allow us to rely more and more on automation – we must be confident that sensing equipment is reporting accurate values. To use the [sanitised] translation of an old adage: ‘rubbish in, rubbish out’.
So, as we leave PID loops in control – we need to be sure that the sensing technology, its connections and ultimately the reading it provides to the control system is reliable.
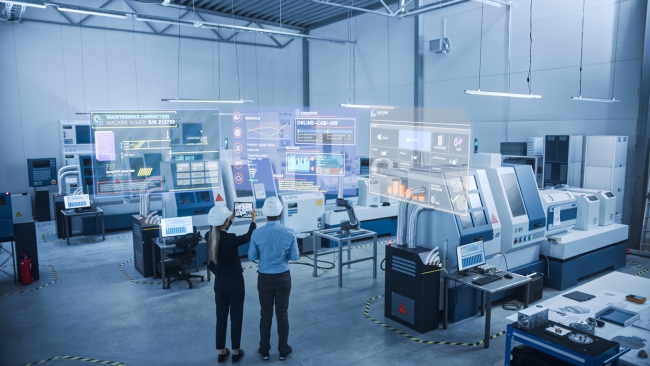
Machine learning can be utilised in many ways, including to predict maintenance events and predict material replenishment activities / Picture: Getty/iStock
Wouldn’t it be nice if we could automatically alert an operator or engineer if sensing equipment is misbehaving?
The good news is this can be done. We recently trained a machine learning model to interpret the signal coming from an existing probe. The model could identify differences in the signal that represented ‘normal’ behaviour, some anomalies that we would expect to see throughout a batch. And, most importantly, it could segregate from both of these, the behaviour that indicated a fault with the probe. The deployment of this early-warning system could detect the issue several days before it was picked up by the operator due to the poor quality of the batch.
What is this information telling our client and what business value could be unearthed? How will they use this timely insight to make more informed decisions about their quality processes?
Machine Learning can help transform your processes by providing real-time predictions to detect unexpected (and expected) quality events whilst empowering corrective action to achieve:
• Real-time process corrections
• Reduced variability rework and rejects
• Giveaway and weight reject management
• Predictive and optimised yield
• Positive pre-release prior to quality inspections
You can learn more about the benefits of machine learning for predictive quality management here.
Utilising Machine Learning to predict material replenishment activities for reduced waste and unplanned downtime
When we look at data from OEE systems, time and time again we see the reason code “Waiting for Materials”.
Ensuring the right materials are in the right place at the right time is critical in avoiding unplanned downtime in your production facility. Finite planning tools can help, and things like low-level alarms on a SCADA can alert us to upcoming shortfalls, but still we see time lost due to waiting for materials.
The problem is that due to on-the-fly changes from production plans, disruption from breakdowns and inconsistencies in material delivery, predicting when a material will need replenished is a tricky business. A low-level alarm or Kanban system can ensure you are requesting materials when they get low – but often materials will arrive too early or too late. If your material runs low, just as you are starting a batch that requires a large quantity – it may run out before the material arrives. If it goes low but is not required for the rest of the shift, you may bring product to it too early. And what happens when two go low at the same time? How do you prioritise the correct replenishment to ensure you avoid any costly delays?
In a recent study of a client’s data, we were able to model the delivery of material from a powder handling unit, allowing us to understand how it is delivered, what tolerances exist and based on the upcoming plan, at what time that material is likely to run out.
This type of model, powered by Machine Learning, gives Cimlogic’s client the predictions required to be proactive rather than reactive when they are planning their replenishment activities. Rather than waiting for a low-level alarm, they can see the order each vessel is likely to run out in, and the amount of time they have before it happens. As you can imagine, this is far more powerful than looking at the remaining level of material in each vessel – especially when working in a facility where each batch has a unique recipe.
A technique like this can save significant amounts of waste through waiting time and unnecessary movements of materials.
What is this information telling our client and what business value could be unearthed? How will they use this timely insight to make more informed decisions about their inventory processes?
Machine Learning can help transform your Inventory and Demand processes by providing real-time predictions to plan material replenishment activities whilst empowering corrective action to achieve:
• WIP optimisation
• Material movement optimisation
• Material availability